A list of my academic publications. See my Google Scholar page for more information.
2023
-
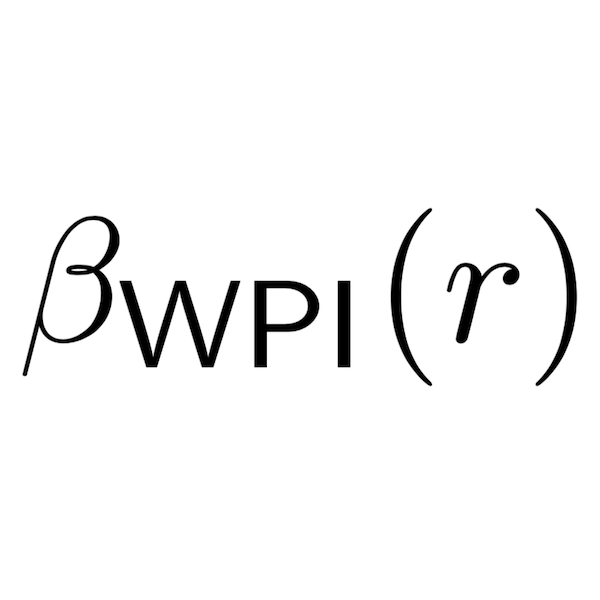
Towards a Complete Analysis of Langevin Monte Carlo: Beyond Poincar\backslash’e Inequality
Alireza Mousavi-Hosseini, Tyler Farghly, Ye He, and 2 more authors
arXiv preprint arXiv:2303.03589 2023
Langevin diffusions are rapidly convergent under appropriate functional inequality assumptions. Hence, it is natural to expect that with additional smoothness conditions to handle the discretization errors, their discretizations like the Langevin Monte Carlo (LMC) converge in a similar fashion. This research program was initiated by Vemapala and Wibisono (2019), who established results under log-Sobolev inequalities. Chewi et al. (2022) extended the results to handle the case of Poincaré inequalities. In this paper, we go beyond Poincaré inequalities, and push this research program to its limit. We do so by establishing upper and lower bounds for Langevin diffusions and LMC under weak Poincaré inequalities that are satisfied by a large class of densities including polynomially-decaying heavy-tailed densities (i.e., Cauchy-type). Our results explicitly quantify the effect of the initializer on the performance of the LMC algorithm. In particular, we show that as the tail goes from sub-Gaussian, to sub-exponential, and finally to Cauchy-like, the dependency on the initial error goes from being logarithmic, to polynomial, and then finally to being exponential. This three-step phase transition is in particular unavoidable as demonstrated by our lower bounds, clearly defining the boundaries of LMC.
-
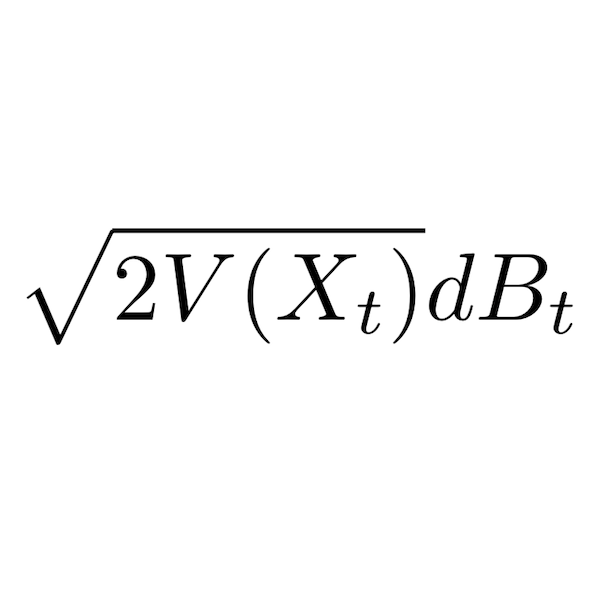
Mean-Square Analysis of Discretized ItĂ´ Diffusions for Heavy-tailed Sampling
Ye He, Tyler Farghly, Krishnakumar Balasubramanian, and 1 more author
arXiv e-prints 2023
We analyze the complexity of sampling from a class of heavy-tailed distributions by discretizing a natural class of Itô diffusions associated with weighted Poincaré inequalities. Based on a mean-square analysis, we establish the iteration complexity for obtaining a sample whose distribution is ϵ close to the target distribution in the Wasserstein-2 metric. In this paper, our results take the mean-square analysis to its limits, i.e., we invariably only require that the target density has finite variance, the minimal requirement for a mean-square analysis. To obtain explicit estimates, we compute upper bounds on certain moments associated with heavy-tailed targets under various assumptions. We also provide similar iteration complexity results for the case where only function evaluations of the unnormalized target density are available by estimating the gradients using a Gaussian smoothing technique. We provide illustrative examples based on the multivariate t-distribution.
2021
-
Time-independent generalization bounds for SGLD in non-convex settings
Tyler Farghly, and Patrick Rebeschini
In Proceedings of Advances in Neural Information Processing Systems 2021
We establish generalization error bounds for stochastic gradient Langevin dynamics (SGLD) with constant learning rate under the assumptions of dissipativity and smoothness, a setting that has received increased attention in the sampling/optimization literature. Unlike existing bounds for SGLD in non-convex settings, ours are time-independent and decay to zero as the sample size increases. Using the framework of uniform stability, we establish time-independent bounds by exploiting the Wasserstein contraction property of the Langevin diffusion, which also allows us to circumvent the need to bound gradients using Lipschitz-like assumptions. Our analysis also supports variants of SGLD that use different discretization methods, incorporate Euclidean projections, or use non-isotropic noise.
2020
-
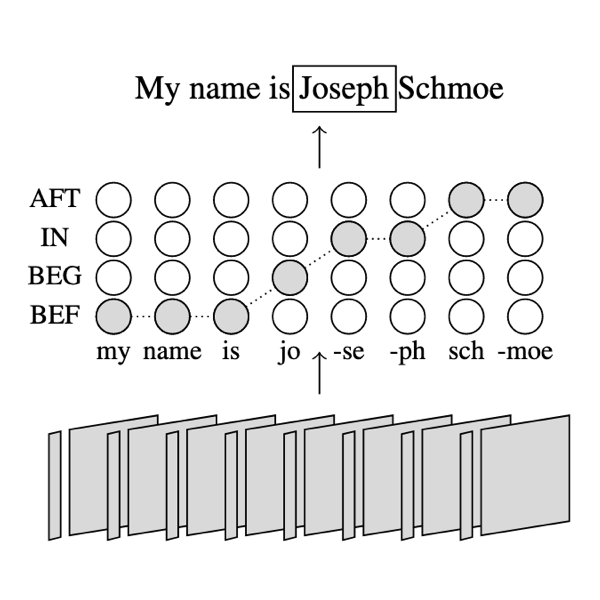
Span-ConveRT: Few-shot Span Extraction for Dialog with Pretrained Conversational Representations
Tyler Farghly, Samuel Coope, Daniela Gerz, and 2 more authors
In Proceedings of the Association for Computational Linguistics 2020
We introduce Span-ConveRT, a light-weight model for dialog slot-filling which frames the task as a turn-based span extraction task. This formulation allows for a simple integration of conversational knowledge coded in large pretrained conversational models such as ConveRT (Henderson et al., 2019). We show that leveraging such knowledge in Span-ConveRT is especially useful for few-shot learning scenarios: we report consistent gains over 1) a span extractor that trains representations from scratch in the target domain, and 2) a BERT-based span extractor. In order to inspire more work on span extraction for the slot-filling task, we also release RESTAURANTS-8K, a new challenging data set of 8,198 utterances, compiled from actual conversations in the restaurant booking domain.